The Rise of Gen AI : Navigating the Future of Artificial Intelligence 🤖
In the dynamic realm of artificial intelligence (AI), few frontiers hold as much excitement and potential for innovation as Generative AI. This cutting-edge field is revolutionizing how we think about creativity, pushing the boundaries of what machines can imagine and create. Join meon a thrilling journey into the world of Generative AI, where imagination knows no limits and innovation knows no bounds.
What is Generative AI?
Generative AI refers to a subset of artificial intelligence techniques focused on generating new data instances that resemble samples from a given dataset. These techniques aim to create new content, such as images, text, audio, or videos, that is similar to the training data but not identical. Generative AI models are capable of producing novel and realistic outputs by learning the underlying patterns and distributions of the training data.
Types of Gen AI models
Generative AI (Gen AI) models can encompass a variety of architectures, techniques, and applications. Here are some types of Gen AI models:
1. Variational Autoencoders (VAEs)
Variational Autoencoders (VAEs) are a class of generative models that leverage an encoder-decoder architecture to map input data into a latent space and reconstruct it back to the original data domain. This architecture aims to balance reconstruction accuracy and regularization to generate new samples that adhere to the learned data distribution.
During the training process, VAEs optimize their parameters to minimize reconstruction error and regularize the distribution of the latent space. This latent space representation facilitates the generation of novel and diverse samples by manipulating points within it.
VAEs find applications in a wide array of domains, including image generation, anomaly detection, and data compression. They enable the creation of realistic images, art synthesis, and interactive exploration of latent spaces.

2. Generative Adversarial Networks (GANs)
Generative Adversarial Networks (GANs) consist of two interconnected networks: a generator and a discriminator. The generator network generates synthetic samples, while the discriminator network distinguishes between real and generated samples in an adversarial manner.
During training, GANs engage in an adversarial game where the generator aims to produce realistic samples to deceive the discriminator, while the discriminator strives to accurately classify samples. Through this adversarial learning process, both networks iteratively improve their performance.
GANs have found numerous real-world applications, particularly in image synthesis tasks such as photorealistic image generation, style transfer, and image inpainting. They have also been applied to text-to-image synthesis, video generation, and virtual environment simulation.

3. Auto-Regressive Models
Auto-Regressive Models generate new samples by modeling the conditional probability of each data point based on the context provided by preceding data points. These models sequentially generate data, allowing for the generation of complex sequences.
During training, auto-regressive models learn to predict the next data point given the previous context. During inference, they generate new samples by sampling from the learned conditional distributions.
Auto-regressive models are commonly used in text generation, language modeling, and music composition tasks. They capture dependencies within sequences and produce coherent and contextually relevant outputs.
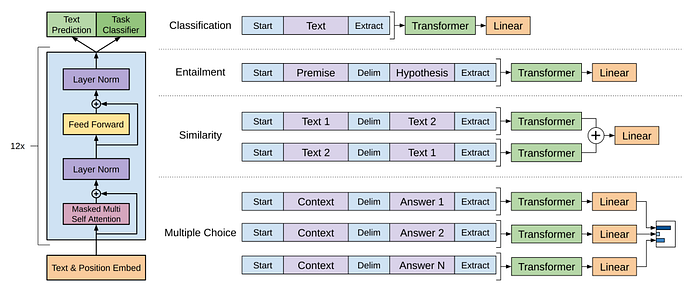
4. Flow-Based Models
Flow-Based Models directly model the data distribution by defining an invertible transformation between the input and output spaces. These models leverage normalizing flows, a sequence of invertible transformations, to model complex data distributions efficiently.
Flow-based models find applications in various domains, including image generation, density estimation, and anomaly detection. They offer advantages such as tractable likelihood evaluation, exact sampling, and flexible latent space modeling.

5. Transformer-Based Models
Transformer-Based Models are a type of deep learning architecture that has gained prominence in natural language processing (NLP) tasks. One notable application of transformer models is the Generative Pre-trained Transformer (GPT) language model, such as GPT-3, which excels in generating coherent and contextually relevant text given a prompt. These models have been utilized for tasks like text completion, question answering, translation, summarization, and more.
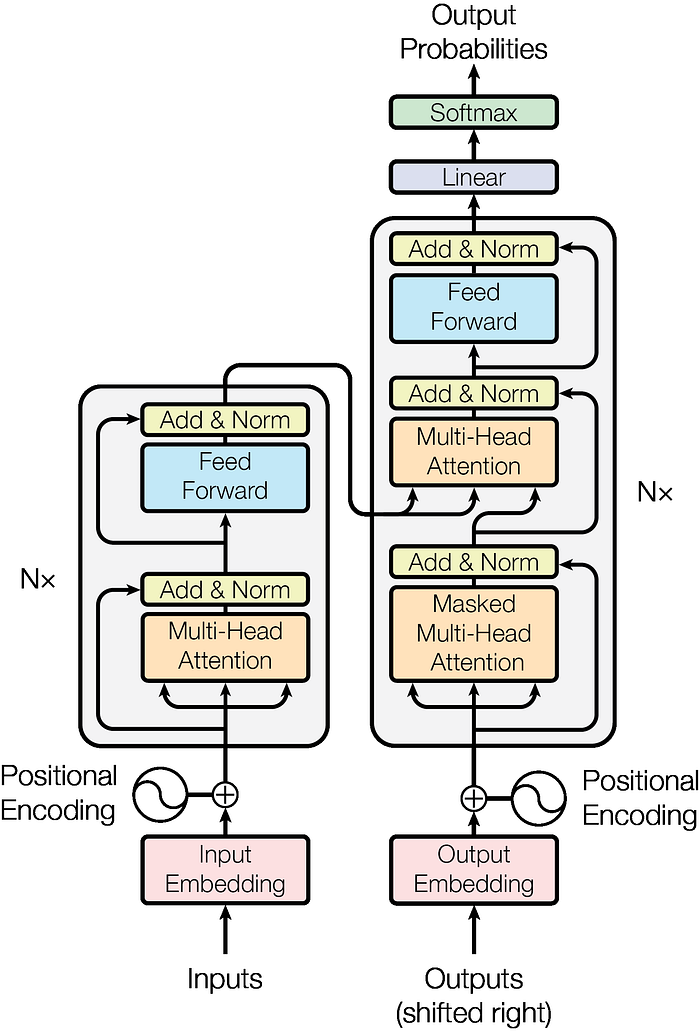
Notable products of Generative AI
Some notable products stemming from Generation AI that have garnered attention include DALL-E, ChatGPT, and BARD.
DALL-E, developed by OpenAI, is a Generation AI model capable of generating unique and imaginative images based on textual descriptions. For instance, given a prompt like “a woman at a music festival twirling her dress, in front of a crowd with glitter falling from the top, long colorful wavy blonde hair, wearing a dress, digital painting,” DALL-E can produce corresponding visual representations.
ChatGPT, another creation by OpenAI, is an AI model specializing in natural and dynamic conversational interactions. It offers intelligent responses across a wide range of topics, engaging users in meaningful and contextually relevant discussions.
BARD, developed by Google, is a language model designed to incorporate Reinforcement Learning techniques. Initially released in response to Microsoft’s integration of GPT into Bing search, BARD aims to enhance language models by interacting with its environment and performing training tasks. Despite some initial setbacks, Google has expanded BARD’s accessibility, marking its presence in over 180 countries and territories.

Applications of Generation AI are diverse and ubiquitous, permeating various facets of daily life. From content generation to data augmentation, virtual reality, gaming, design, music composition, medical imaging, language translation, financial modeling, and more, Generation AI finds utility across numerous fields, shaping the way we interact with technology and fostering innovation in countless domains.
Applications Across Domains
Generative AI finds applications across a wide range of domains, unleashing creativity and innovation in diverse fields:
- Art and Design: Generative AI enables artists and designers to explore new creative possibilities, generating unique artworks, designs, and visualizations. From abstract paintings to intricate digital sculptures, Generative AI sparks new forms of artistic expression.
- Content Generation: In the realm of content creation, Generative AI revolutionizes storytelling, music composition, and video production. AI-generated text can fuel storytelling engines, while music composition algorithms produce original compositions tailored to specific moods or genres.
- Data Augmentation: Generative AI plays a crucial role in data augmentation, enhancing training datasets for machine learning models. By generating synthetic data samples, Generative AI helps improve model performance and generalization across various tasks, from image recognition to natural language processing.
- Scientific Discovery: In scientific research, Generative AI aids in drug discovery, molecular design, and materials science. By generating novel molecular structures or predicting material properties, Generative AI accelerates the pace of innovation and discovery in fields like pharmaceuticals and materials engineering.
The Future of Creativity
As Generative AI continues to advance, its potential for creativity and innovation knows no bounds. From generating lifelike images to composing original music, Generative AI opens up new frontiers of exploration and discovery, pushing the boundaries of human imagination.
In the hands of artists, researchers, and creators, Generative AI serves as a powerful tool for unlocking new forms of expression, enabling collaboration across disciplines, and shaping the future of creativity in profound ways. As we embark on this journey of exploration, let us embrace the transformative potential of Generative AI to inspire, amaze, and delight the world.